- Blog
Why IoT and Big Data Must Be Interconnected
Learn why IoT and Big Data must be interconnected to unlock real-time insights, enhance decision-making, and drive innovation.
The digital world is increasingly interconnected, driven by two powerful forces: the Internet of Things (IoT) and Big Data.
The Internet of Things (IoT) refers to the vast network of physical objects embedded with sensors, software, and other technologies that connect and exchange data over the internet with other devices and systems. From smart home devices and wearable tech to industrial sensors and connected vehicles, IoT is transforming how we interact with our environment and collect information.
Big Data, on the other hand, refers to extremely large and complex datasets that cannot be easily processed or analyzed by traditional data processing applications. It’s characterized by the “Five Vs”: Volume, Velocity, Variety, Veracity, and Value.
How IoT Impacts Big Data
IoT has a profound and direct impact on every aspect of Big Data:
- Massive Increase in Data Volume: IoT devices generate an unprecedented amount of data. Every sensor reading, every status update, and every interaction contributes to an explosion in data volume, pushing the boundaries of what constitutes “big.”
- Greater Data Variety: The data generated by IoT devices comes in many forms. It includes structured sensor readings, semi-structured logs, and unstructured data like images and video from smart cameras. This diverse range of data types adds significant complexity to Big Data environments.
- Accelerated Data Velocity: Many IoT applications demand real-time or near-real-time processing. Think of autonomous vehicles needing instant sensor data to make decisions, or industrial machinery requiring immediate anomaly detection. IoT dramatically increases the speed at which data is generated and needs to be analyzed.
- New Data Characteristics (Veracity & Variability):
- Veracity: IoT data can be prone to errors due to sensor malfunctions, environmental interference, or network issues, challenging data quality and reliability.
- Variability: The rate and format of data streams from IoT devices can change dynamically, requiring flexible and adaptive Big Data processing solutions.
In essence, IoT acts as a massive data generator, continuously feeding Big Data ecosystems with more, faster, and more diverse information. This symbiotic relationship means that while IoT creates the need for Big Data solutions, Big Data technologies are essential for extracting meaningful insights and value from the vast amounts of data that IoT produces.
The integration of IoT and Big Data delivers measurable business results, with the majority of companies implementing IoT projects reporting increased revenue. As connected devices continue to generate massive data volumes, data professionals now face the critical task of converting these information streams into strategic actions. Companies that successfully combine IoT and Big Data analytics gain significant advantages through predictive maintenance capabilities, continuous monitoring systems, and data-driven decision automation.
This article provides practical implementation strategies for IoT and Big Data solutions in the manufacturing, healthcare, and supply chain sectors, complete with solutions to common technical obstacles. You’ll learn specific steps to transform raw IoT data into valuable business intelligence that drives growth and operational efficiency.
Practical Applications of Data Visualization for IoT and Big Data Analytics
When IoT devices and Big Data analytics combine forces, they create tangible business value across multiple sectors. Let’s examine how organizations are implementing these technologies to solve real business challenges and drive measurable improvements in operations, customer service, and profitability.
Predictive Maintenance: Preventing Failures Before They Happen
Predictive maintenance represents one of the most valuable applications of IoT and Big Data analytics. Through continuous monitoring of equipment performance data, organizations can identify patterns that precede failures and schedule maintenance activities precisely when needed—not too early (wasting resources) and not too late (causing breakdowns).
The formula for successful predictive maintenance implementation includes:
- Sensor selection and placement: Identifying critical measurement points and installing appropriate sensors (vibration, temperature, acoustics, etc.)
- Data architecture creation: Building systems that can handle high-frequency sensor readings and store historical performance data
- Pattern recognition: Using machine learning algorithms to identify abnormal operating conditions that precede failures
- Integration with maintenance workflows: Connecting analytics insights to work order systems and maintenance teams
Supply Chain Visibility and Optimization
Supply chain operations benefit significantly from IoT and Big Data integration. Smart sensors attached to shipments track location, temperature, humidity, and handling conditions in real time, while Big Data analytics platforms process this information to optimize routing, predict delays, and ensure product quality.
Healthcare Monitoring and Patient Care Improvements
Healthcare providers are leveraging IoT and Big Data analytics to improve patient outcomes while reducing costs. Remote patient monitoring devices collect vital signs, medication adherence data, and activity levels, while analytics platforms identify concerning patterns that require intervention.
Challenges and Solutions in IoT and Big Data Implementation
While the potential of IoT and Big Data is immense, organizations often encounter significant hurdles when implementing these technologies. Understanding these challenges and having practical strategies to address them is essential for successful deployment.
Real-Time Data Visualization Platform for
IoTLife SciencesData LakesManufacturing
-
Interactive 3D Models
Add relevant context such as floor plans, technical drawings, and maps
-
Semantic Zoom Capability
Effortlessly navigate and drill-down between different levels of detail
-
Configurable Dashboards
Design visualizations exactly how you’d like to see them
Data Security and Privacy Considerations
IoT ecosystems create expanded attack surfaces, with each connected device potentially serving as an entry point for security breaches. To mitigate these risks, organizations need comprehensive security strategies specifically designed for IoT environments. These strategies must address device authentication, data encryption, and secure network architecture:
- Implement device authentication protocols: Ensure that each IoT device has unique identity credentials and cannot connect to your network without proper verification.
- Deploy end-to-end encryption: Secure data both at rest and in transit using industry-standard encryption methods.
- Establish network segmentation: Isolate IoT devices from critical business systems using VLANs or similar technologies.
- Conduct regular security audits: Schedule automatic vulnerability scans and penetration testing specifically targeting IoT infrastructure.
- Develop incident response plans: Create detailed procedures for addressing security breaches when they occur.
Following these steps creates multiple layers of protection that significantly reduce the likelihood of security incidents while maintaining compliance with data protection regulations.
Scalability and Infrastructure Requirements
As IoT deployments expand, organizations often struggle with scaling their infrastructure to handle growing data volumes. Traditional data centers typically lack the flexibility needed for IoT workloads, which can fluctuate dramatically based on usage patterns.
Cloud-based platforms provide the flexibility and scalability necessary for IoT implementations, with services like AWS IoT and Azure IoT Hub offering purpose-built solutions. These platforms automatically scale computing resources based on current demand, ensuring optimal performance without unnecessary costs.
Data Quality and Standardization Issues
IoT data frequently arrives in inconsistent formats from diverse devices, creating significant challenges for meaningful analysis. Without standardization, organizations struggle to integrate data streams or draw accurate conclusions.
Effective data quality management requires implementing data governance frameworks that establish clear standards for data collection, processing, and storage. Organizations should develop data dictionaries that define common terms and measurement units across all IoT systems to ensure consistency in analysis.
Implementing Effective Analytics Strategies
Many organizations collect vast amounts of IoT data but struggle to extract meaningful insights. The majority of IoT projects stall at the proof of concept stage because they lack clear analytics strategies tied to business outcomes.
Success requires starting with specific business questions, then working backward to determine what data is needed and how it should be analyzed. Organizations should focus on creating purpose-built analytics pipelines that process only the most relevant data points rather than attempting to analyze everything.
Optimizing Your IoT and Big Data Strategy with Hopara
Implementing successful IoT and Big Data analytics requires specialized tools that can manage complex data streams and transform them into actionable business intelligence. Let’s examine how Hopara’s visualization platform addresses the specific challenges faced by data professionals working with IoT data.
How Hopara Transforms Raw IoT Data into Actionable Insights
The gap between collecting IoT data and deriving value from it remains substantial for many organizations. Companies typically use only a small fraction of IoT data for decision-making, leaving tremendous potential untapped.
Hopara bridges this gap through an intuitive data exploration system that processes complex IoT data streams and presents them in comprehensible visual formats. The platform uses advanced data processing algorithms to handle time-series data from sensors and connected devices, automatically identifying patterns and anomalies that might otherwise remain hidden.
Real-Time Visualization Capabilities for IoT Data Streams
Effective IoT monitoring requires near-instantaneous visualization of streaming data. Hopara processes incoming data feeds with minimal latency, enabling operations teams to react immediately to changing conditions.
Feature | Traditional BI Tools | Basic IoT Dashboards | Hopara Platform |
---|---|---|---|
Refresh Rate | Daily/Hourly | Minutes | Seconds / Real-Time |
Data Volume Capacity | Limited | Medium | High (billions of records) |
Anomaly Detection | Manual | Basic Thresholds | AI-Powered Pattern Recognition |
User Technical Requirements | High | Medium | Low (Accessible to Non-Technical Users) |
Integration with Existing IoT Infrastructure and Data Sources
The value of IoT data multiplies when combined with contextual information from other business systems, creating a comprehensive operational picture that drives more informed decisions. Hopara connects seamlessly with diverse data sources, including industrial sensors, MQTT brokers, time-series databases, and enterprise systems. This integration capability allows organizations to create unified dashboards that combine IoT telemetry with business metrics, providing context that enhances decision-making.
Customizable Analytics for Industry-Specific IoT Applications
Industries have different requirements for IoT data analysis. Manufacturing facilities focus on equipment efficiency metrics, while pharmaceutical labs monitor environmental conditions and quality parameters. Hopara offers industry-specific templates and customizable dashboards that address these specialized needs without requiring extensive configuration.
For pharmaceutical labs, the platform provides FDA 21 CFR Part 11 compliant data handling with audit trails and electronic signatures. In manufacturing environments, it connects directly to PLCs and SCADA systems to monitor production metrics and equipment performance. These purpose-built solutions accelerate implementation and deliver faster time to value. Contact us to explore how our customizable analytics can be tailored to your specific industry requirements.
Conclusion: Maximizing Value from IoT and Big Data Analytics
The combination of IoT and Big Data represents a transformational opportunity for organizations that can effectively harness their synergy. Companies that implement integrated approaches see 3-5x greater ROI on their technology investments than those treating these technologies in isolation.
When organizations properly integrate IoT and Big Data analytics, they create a robust foundation for business intelligence that shifts operations from reactive to predictive models. Companies achieve substantial value through systematic approaches to implementation challenges while maintaining focus on specific business outcomes. This leads to meaningful improvements in operational efficiency, customer experience quality, and opportunities for new service development.
Contact us today to discover how Hopara’s visualization platform can help your team extract maximum value from your IoT data streams while simplifying complex analytics for both technical and business stakeholders.
Real-Time Data Visualization Platform for
IoTLife SciencesData LakesManufacturing
-
Interactive 3D Models
Add relevant context such as floor plans, technical drawings, and maps
-
Semantic Zoom Capability
Effortlessly navigate and drill-down between different levels of detail
-
Configurable Dashboards
Design visualizations exactly how you’d like to see them
FAQs
What are the security best practices for implementing IoT and Big Data analytics systems?
When setting up Big Data and IoT solutions, companies should focus on layered security measures. Start with device-specific authentication certificates, then implement full encryption across all data transfers. Make sure to separate IoT networks from essential business systems and set up automated security scanning tools specifically designed for IoT environments. Your security team should run thorough checks of your IoT and Big Data analytics infrastructure every three months to catch new weaknesses that might appear as your system grows.
How does the integration of IoT and Big Data improve financial outcomes for businesses?
Mainly through better operations, reduced maintenance expenses, and fresh income sources. Most organizations experience lower maintenance costs thanks to predictive analytics that help avoid expensive equipment breakdowns. Companies also report improvements in production efficiency from adjustments based on real-time sensor data.
What data storage architecture works best for handling massive IoT and Big Data volumes?
Managing huge IoT and Big Data volumes requires a layered storage approach for best results and cost management. This typically combines hot storage using time-series databases for fresh, frequently-needed data; warm storage in columnar databases for mid-term analysis; and cold storage in distributed file systems for long-term records and deep analysis. Smart companies create automatic data movement rules that shift information between these layers based on age and usage patterns, helping balance performance needs against storage costs as IoT data continues to grow.
How can non-technical teams extract value from IoT and Big Data without specialized data science knowledge?
Tools like Hopara make Big Data and IoT insights accessible to everyone through straightforward dashboards that don’t require technical skills. These platforms let users ask questions in everyday language instead of writing complicated code or database queries. Ready-made visualization templates for different industries allow operations staff, business analysts, and executives to explore IoT data connections on their own. This speeds up decision-making and reduces the need to have specialized data scientists at every step.
What implementation timeline should companies expect for enterprise-scale IoT and Big Data analytics projects?
Large-scale IoT and Big Data analytics projects usually take 6-18 months from initial planning to complete deployment, depending on company size and existing systems. The process typically follows four main stages: 1-2 months for discovery and planning, 2-3 months for a small test deployment with limited sensors, 3-6 months for broader rollout, and 2-4 months fine-tuning analytics models and dashboards. Companies that use a step-by-step approach focusing on quick results tend to see faster returns on their IoT and Big Data investments than those trying to deploy everything at once.
Want to learn more how Hopara can help you?
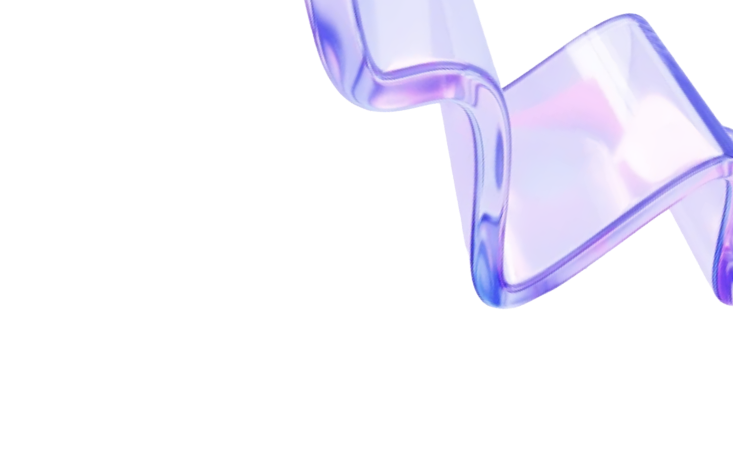
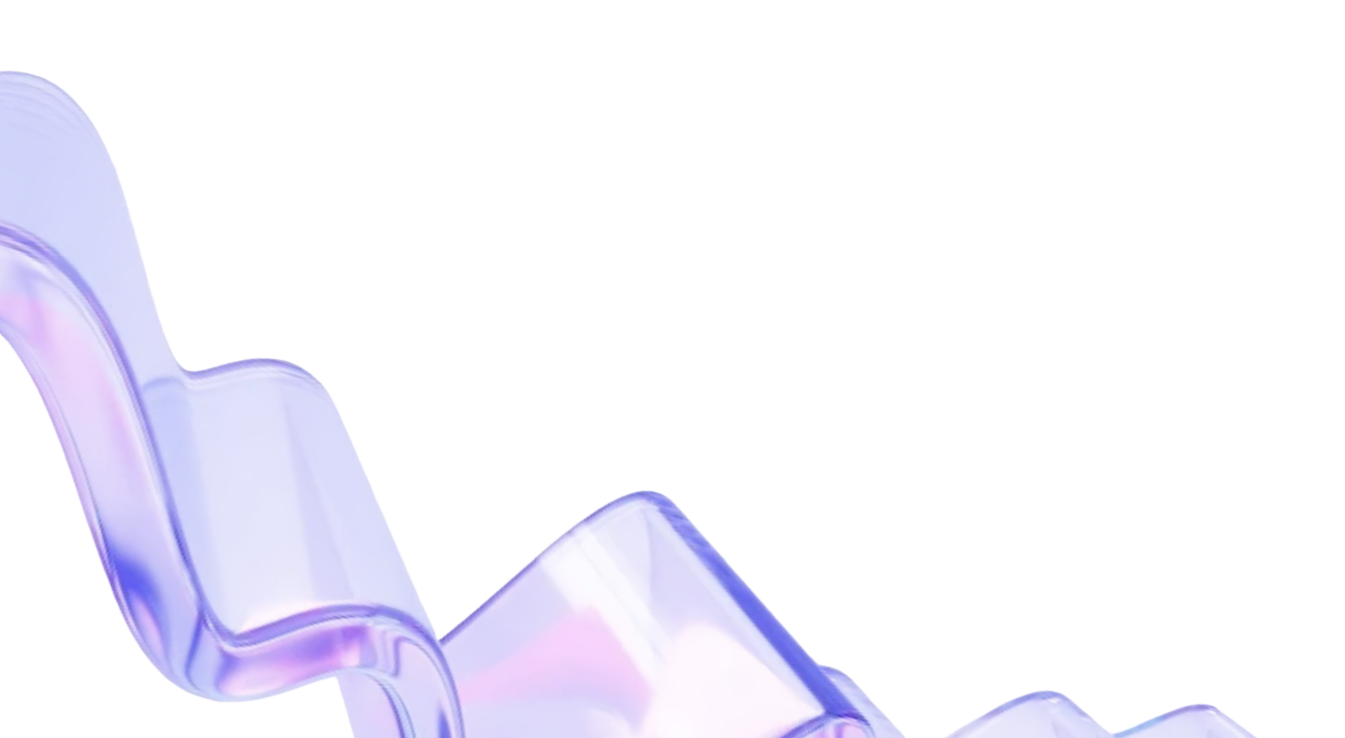